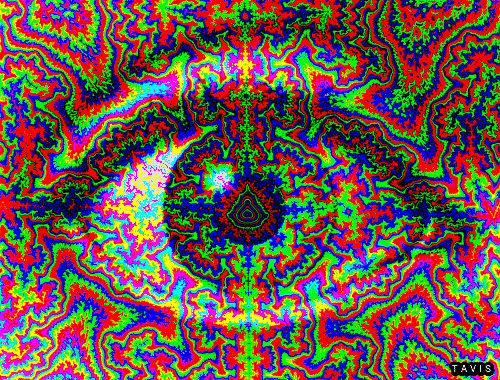

I have been using deephermes daily. I think CoT reasoning is so awesome and such a game changer! It really helps the model give better answers especially for hard logical problems. But I don’t want it all the time especially on an already slow model. Being able to turn it on and off wirhout switching models is awesome. Mistral 24b deephermes is relatively uncensored, powerful and not painfully slow on my hardware. a high quant of llama 3.1 8b deephermes is able to fit entirely on my 8gb vram.
You are correct in your understanding. However the last part of your comment needs a big asterisk. Its important to consider quantization.
The full f16 deepseek r1 gguf from unsloth requires 1.34tb of ram. Good luck getting the ram sticks and channels for that.
The q4_km mid range quant is 404gb which would theoretically fit inside 512gb of ram with leftover room for context.
512gb of ram is still a lot, theoretical you could run a lower quant of r1 with 256gb of ram. Not super desirable but totally doable.